With the greatest potential upside from adopting artificial intelligence and analytics across all sectors, the retail industry is in the midst of a significant transformation. Many retailers view AI as indispensable to a robust omnichannel strategy and believe it can drive the highest revenue growth within e-commerce. Indeed, in the contemporary retail landscape, AI is already playing an instrumental role in enhancing operational efficiency, boosting/diversifying revenue streams, and improving customer experience through the utilization of Retail Media Networks (RMNs). In this article, we shed light on how RMNs leverage AI to grant retailers a competitive advantage; underscore real-world success stories; and elaborate on some of the common challenges to AI implementation within retail.
AI in Retail Media: Three Pillars of Business Value
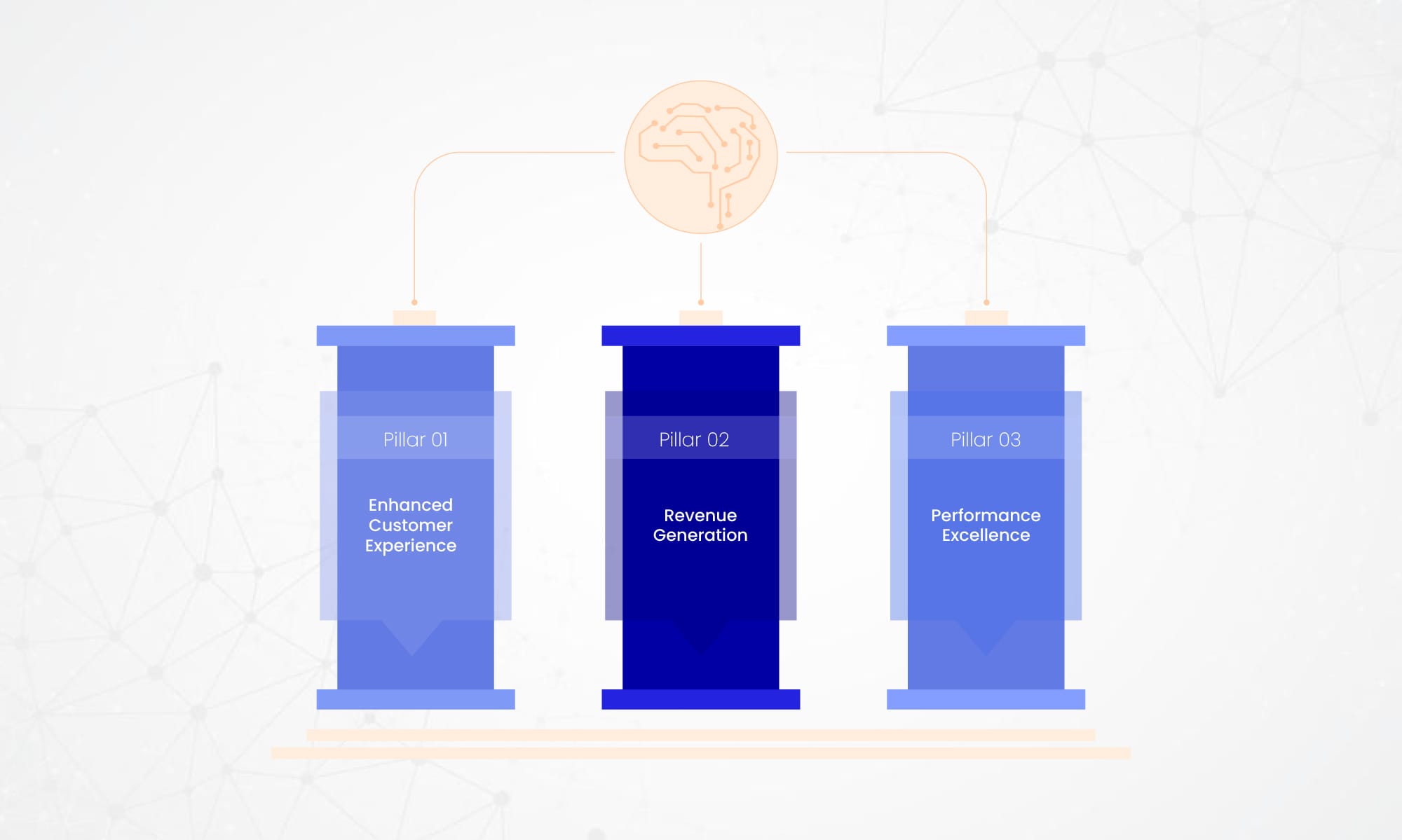
Enhanced Customer Experience: The AI Advantage
In today's data-driven retail landscape, AI has become instrumental in delivering personalized customer experiences that drive engagement and conversions. For advanced audience segmentation, machine learning models process and analyze multiple data streams simultaneously - including historical purchase data, browsing behavior, interaction patterns, and demographic information. These algorithms employ sophisticated clustering techniques and predictive modeling to identify micro-segments and correlation patterns that would be impossible to detect manually. In doing so, these models enable retailers to create highly targeted customer segments that account for not just historic but current customer behaviors and preferences.
Dynamic Creative Optimization (DCO)
Represents another powerful application of AI in retail media. The technology employs a modular creative approach, deconstructing advertisements into individual components - from headlines and images to call-to-action buttons - which are stored in dynamic creative libraries. As users interact with digital content, AI models analyze behavioral signals, contextual data, and performance metrics to determine optimal creative combinations. This automated optimization process leverages multivariate testing and machine learning algorithms to assemble and serve personalized ad variations in milliseconds, continuously refining performance over time.
Furthermore, AI's role in personalized ad delivery extends beyond simple targeting. Modern AI systems can predict optimal timing and placement for advertisements, ensuring they reach customers when they're most likely to engage. By continuously learning from customer interactions and campaign performance data, these systems refine their targeting capabilities over time, leading to increasingly effective personalization strategies that enhance both customer satisfaction and campaign ROI.
Smart Bidding and Inventory Management
The integration of AI in retail media networks has revolutionized how retailers approach advertising spend and inventory management. By leveraging advanced AI systems, retailers can now synchronize their advertising efforts with real-time inventory data, ensuring that marketing budgets are allocated efficiently and effectively. In doing so, retailers avoid the common pitfall of promoting out-of-stock items, while simultaneously optimizing ad delivery for available products.
AI's ability to process vast amounts of customer data within RMNs has transformed the landscape of inventory-aware advertising. The technology continuously monitors stock levels, automatically adjusting campaign parameters and ad messaging to align with product availability. This dynamic approach not only minimizes wasted ad spend but also enables retailers to implement sophisticated strategies, such as targeting specific customer segments with value-based messaging when inventory patterns suggest shifting consumer behaviors.
The impact of this AI-driven synchronization extends beyond immediate cost savings. AI systems within RMNs leverage customer data to provide actionable insights that inform strategic decision-making. This comprehensive approach to data analysis enables retailers to build a more complete picture of their advertising effectiveness, leading to improved ROI and more strategic resource allocation across their retail media initiatives.
Performance Excellence: The Power of Predictive Analytics
In the evolving landscape of retail media, AI's predictive capabilities have fundamentally transformed how campaigns are conceived, executed, and optimized. Rather than relying on traditional guesswork or basic demographic targeting, AI systems can now analyze millions of data points from previous campaigns to predict performance outcomes before launch. This shift from reactive to predictive campaign management represents a significant leap forward in advertising effectiveness.
The real power of AI-driven predictive analytics lies in its ability to identify and interpret complex patterns in customer behavior. By processing vast amounts of proprietary campaign data, AI systems can forecast consumer actions with unprecedented accuracy - from determining which marketing messages will resonate with specific audiences to predicting optimal timing for ad delivery. These insights enable retailers to create highly targeted campaigns that adapt to shifting consumer behaviors in real-time, significantly improving both efficiency and cost-effectiveness.
Success Stories: Transforming Retail Media with AI
Leveraging customer data to boost revenue
Leading retailers are already demonstrating the transformative impact of AI in retail media, particularly in the realm of targeted advertising. Walmart, a pioneer in retail innovation, has successfully implemented AI-driven analysis across its digital and physical channels to revolutionize ad delivery. By processing and analyzing vast amounts of customer data, Walmart's AI systems create more precise targeting parameters, ensuring that customers encounter relevant advertisements throughout their shopping journey. This sophisticated approach to ad placement has not only enhanced customer engagement but has also delivered measurable improvements in ROI, showcasing the tangible business impact of AI-powered targeting.
CVS Health
Presents another compelling example of AI's potential in retail media. Through its RMN (CVS Media Exchange) the pharmacy giant has created an integrated advertising ecosystem that spans digital touchpoints - from its website and mobile app to email communications and in-store displays. What sets CVS's approach apart is its strategic use of first-party data collected from over 9,900 locations. By feeding this rich customer data into AI algorithms, CVS creates highly targeted customer segments, enabling more precise ad delivery and personalized shopping experiences. This comprehensive approach to AI-powered retail media has proven particularly effective, driving significant improvements in both customer engagement and revenue generation.
Challenges in AI Implementation within Retail
Data Quality Requirements
The effectiveness of AI systems in retail media hinges critically on the quality and quantity of available data. While retailers generate vast amounts of customer information daily, transforming this raw data into actionable insights presents significant challenges. Poor data quality, inconsistencies across sources, or incomplete datasets can severely compromise AI's predictive capabilities, leading to flawed marketing strategies and misallocated resources.
Personnel and Technical Expertise
The competitive demand for AI specialists and data scientists poses a substantial hurdle for retailers implementing AI solutions. Beyond initial implementation, these experts are crucial for ongoing system maintenance, optimization, and innovation. The scarcity of qualified professionals has emerged as a primary barrier to AI adoption, particularly affecting retailers who lack the resources to compete for top talent in an increasingly competitive market.
Legacy Infrastructure Challenges
Many retailers struggle with outdated technology infrastructure that impedes the seamless integration of AI systems. These legacy systems often result in fragmented data silos, limiting retailers' ability to leverage AI effectively across critical initiatives like demand forecasting and inventory management. The challenge extends beyond mere technical limitations - it impacts the scalability and effectiveness of AI implementations across the retail organization.
Investment and Optimization Costs
The financial implications of implementing AI systems extend well beyond initial setup costs. Retailers must invest significantly in data infrastructure, specialized software, and ongoing system maintenance. Many AI projects stall during implementation due to unexpected costs and integration complexities. This challenge is particularly acute for smaller retailers, who must balance the promise of AI capabilities against substantial upfront and ongoing investments.
The Path Forward: Balancing Innovation with Implementation
As the retail landscape continues to evolve, the adoption of AI technologies isn’t optional — it's a competitive necessity. Industry data reveals an emerging consensus around a hybrid approach to AI adoption, with 52 percent of retailers choosing to balance internal control with external expertise. This trend is even more pronounced among larger retailers, where 63 percent of those with annual revenues exceeding $500 million opt for collaborative implementation strategies.
This hybrid approach offers a pragmatic solution, enabling retailers to navigate the complexities of AI adoption while maintaining strategic control. By partnering with experienced technology providers, organizations can address key challenges — from technical expertise gaps to infrastructure limitations — while building AI-driven retail media capabilities that create more personalized, efficient, and profitable advertising ecosystems.
Want to know how? Try Carter